AI is considered a savior for improving efficiency and overall performance, unlocking new sales potential, and evolving business models. Top management, in particular, have high expectations for the use of generative AI within their own organization. Budgets for AI projects have been generously increased, as our study shows, and an entire catalog of measures and initiatives for 2024 has been enthusiastically approved.
However, the expected benefits associated with adopting these new technologies will not materialize in many companies. The reason: there is a lack of strategic prioritization, budgets are not allocated reasonably. The measures are not managed holistically, and implementation often does not follow a tried-and-tested approach. The effort and challenges of implementation are often overlooked.
Scattergun approach burns budgets and resources
Currently, the majority of companies are following the scattergun approach, as revealed by our study. Following the motto “a lot helps a lot”, they initiate AI measures in every department and conceivable category. All measures are approached with (almost) the same priority—the budget is distributed accordingly in a granular manner. The same applies to internal resources. Operational challenges and risks are significantly underestimated. Departments are already groaning and criticize the lack of strategic positioning, complain about insufficient internal expertise and the shortage of specialized team members.
To illustrate: If I have only limited building materials and craftsmen, yet I open ten construction sites, at most two will be completed within the planned timeframe. Instead of a sustainable, self-sustaining property on a solid foundation with expansion potential that appreciates in value, the result is a run-of-the-mill building with planning errors, conventionally operated—and now we’re already talking about developing the next new residential area. In short, many companies will run out of financial steam before they can even embark on the next level of AI transformation. By then, the competition will have long surpassed them.
Copycat AI—simply transfer the proven approach
What can help? Following a proven approach model—especially when it comes to the dynamic trending topic of AI. Because AI transformation is also a digital transformation, and it is highly controllable and predictable. The recipes for success of digital transformation can be almost directly applied to AI. Sounds practical and synergistic? That's exactly the case.
1. Potential benefit analysis
No, there’s no way around this basic work. And yes, it’s still worthwhile even if various individual measures and projects are already underway or approved. What should be included? The current company status (strategy, business model, business areas, revenue development, etc.). This is contrasted with (Gen)AI potential as a whole—and then analyzed using classical methods, as open-ended as possible, and with interdisciplinary teams. Currently, only a quarter of companies have implemented such an analysis or are currently implementing it. For 2024, another 50 percent have committed to thoroughly examining potential use cases—we didn’t inquire about the depth or exact timing. Let’s hope this measure isn’t sacrificed in favor of perceived and exciting quick wins.
2. Strategy
Based on the analysis results, a fundamental strategy for the company’s own AI transformation is developed. This includes: concrete goals and priorities, operating model, governance framework, use case portfolio, handling uncertainties, enablement, communication, and of course, a timeline. The overall budget is allocated to selected business areas and use cases. Similarly, only one in four companies state that they have already set the right strategic course. For another 50 percent, the roadmap is on the agenda for 2024—after all.
In any case, less is more! Because even before the actual implementation, extensive prerequisites need to be met and preparatory work needs to be done, especially regarding data base and data quality. Ethical and regulatory fundamental questions also need to be clarified in advance.
3. Suitable implementation approach
Various archetypes of AI implementation have already proven themselves in companies. These include, firstly, the “sandbox approach" or "try-out approach", in which AI is tested in a protected environment. Secondly, there is a structured analytical approach along the business processes and thirdly, the "AI all-in approach", in which GenAI is applied for scaled use in the company.
4. Gradual implementation
The actual implementation proceeds incrementally with close monitoring and ongoing adjustments. Proof of concepts should be repeatedly tested in sandboxes. Caution: Establish transparency within the company from the outset regarding what precisely is happening and what is planned concerning the use of AI. Use all available internal communication channels to provide timely information about project plans and updates.
5. Empowering and motivating employees Even the most expensive AI is useless if employees do not use it. Analyze the existing know-how and plan a roadmap for employee training and development. Appoint pilot project leads and turn those affected into participants.
If this all sounds too much like a template, it should be noted that, depending on the company, different priorities can of course be set in the in-house "road to AI transformation".
Navigating the AI revolution without a plan, with blind actionism or preconceived, untested notions, hoping that the AI spark will somehow ignite throughout the company, is definitely the inferior alternative.
Your Contact
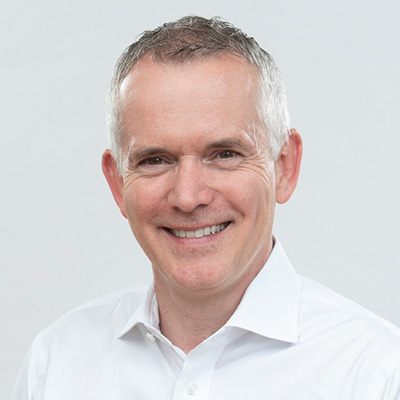
Rainer Zierhofer
Horváth Study
Mastering the AI revolution with a competitive edge - what CxOs need to know now

Interview with Bernd Wagner, Managing Director of Google Cloud in Germany
"AI-optimized products and services will become commodities – and no company will be able to afford falling behind"
